|
Research highlight : CIDR 2015: INVISIBLE GLUE: SCALABLE SELF-TUNING MULTI-STORES |
 |
 |
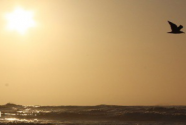 |
 |
CIDR 2015: INVISIBLE GLUE: SCALABLE SELF-TUNING MULTI-STORES
24 October 2014
Francesca Bugiotti, Damian Bursztyn, Alin Deutsch, Ioana Ileana and Ioana Manolescu
|
Next-generation data centric applications often involve diverse datasets, some very large while others may be of moderate size, some highly structured (eg, relations) while others may have more complex structure (eg, graphs) or little structure (eg, text or log data). Facing them is a variety of storage systems, each of which can host some of the datasets (possibly after some data migration), but none of which is likely to be best for all, at all times.
Deploying and efficiently running data-centric applications in such a complex setting is very challenging.
We propose Estocada, an architecture for efficiently handling highly heterogeneous datasets based on a dynamic set of potentially very different data stores. Estocada provides to the application/programming layer access to each data set in its native format, while hosting them internally in a set of potentially overlapping fragments, possibly distributing (fragments of) each dataset across heterogeneous stores. Given workload information, Estocada self-tunes for performance, ie it automatically choses the fragments of each data set to be deployed in each store so as to optimize performance. At the core of Estocada lie powerful view-based rewriting and view selection algorithms, required in order to correctly handle the features (nesting, keys, constraints etc) of the diverse data models involved, and thus to marry correctness with high performance.
Keyword
[none]
Group
[none]
Contact
[none]
|
 |  |
|
|
 |
|